Multi-source K-nearest neighbor, Mean Balanced forest inventory of Georgia
Abstract
We describe here a case study in compiling a high-resolution forest inventory for central Georgia using the K-nearest neighbor approach with multi-source data and Mean Balancing correction for the estimation bias. In general, multi-source data collected through various incompatible designs cannot be mixed due to intractable variances and unknown bias. Because of this incompatibility abundant information about the environment (i.e. atmospheric conditions, soil composition, spatio-temporal data from nearly 40 years of satellite imaging, and a wealth of site specific studies with sampling for various growth attributes) frequently cannot be used to produce new unbiased estimates for the variables and areas of interest. This study was carried out in central Georgia, and the k-NN approach was used to fuse together various incompatible data from public and private sources. We used the Mean Balancing approach to remove the bias resulting from this data fusion. The result of the study is a derivation of an unbiased high-resolution forest inventory, which can be used for small area's fiber supply assessment analysis.
Keywords
Refbacks
- There are currently no refbacks.

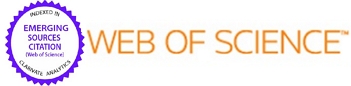
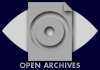
© 2008 Mathematical and Computational Forestry & Natural-Resource Sciences