Adjacency constraints in forestry – a simulated annealing approach comparing different candidate solution generators
Abstract
Adjacency constraints along with harvest volume constraints are important in long term forest management planning. Simulated annealing (SA) has previously been successfully applied when addressing such constraints. The objective of this paper is to assess the performance of SA using three methods for generating candidate solutions. Biased probabilities in the management unit (MU) selection were introduced, one static and one dynamic. The rst one (Method 1) is the conventional (static) method. The two other methods were implemented through a search vector used in the candidate solution generator. These methods are based on (Method 2) the number of treatment schedules and standard deviation of NPV within MUs and (Method 3) the MU's potential improvement in the objective function value, the number of URM adjacency violations an MU is involved in, the period specic volume harvested in an MU and the number of times an MU is selected. The methods were tested on a large number of datasets including 300 hypothetical forest landscapes characterized by three dierent initial age class distributions, respectively young, normal and old. Evaluation of the methods was accomplished by means of objective function values and rst feasible iteration. Solutions improved when introducing bias in the probabilities for MU selection (Methods 2 and 3) compared to the conventional method (Method 1) and when the probability bias for selecting MUs is dynamic (Method 3) rather than static (Methods 1 and 2). The mean improvement for the average GAP obtained by Method 3 for young, normal and old forest landscapes was 20.88%, 12.84% and 5.20%, respectively. Whereas for the minimum GAP the mean improvement was 21.96%, 14.30% and 6.05% for young, normal and old forest landscapes, respectively.
Keywords
Static and dynamic search vectors; grid landscapes; heuristics; Unit restriction model
Full Text:
PDFRefbacks
- There are currently no refbacks.

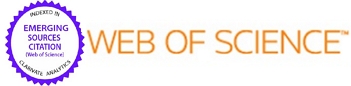
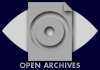
© 2008 Mathematical and Computational Forestry & Natural-Resource Sciences