ALTERNATE RANKED SET SAMPLING FOR SKEWED AND MOUND SHAPED SYMMETRIC DISTRIBUTIONS: ACCOUNTING FOR FORESTRY AND ENVIRONMENTAL RESEARCH
Abstract
Keywords
Full Text:
PDFReferences
Acharya, B., Bhattarai, G., Gier, A.D. and Stein, A. (2000). Systematic adaptive cluster sampling for the assessment of rare tree species in Nepal. Forest Ecology and Management, 137(1-3), 65-73.
Barnett, V. (1999). Ranked set sample design for environmental investigations. Environmental and Ecological Statistics, 6, 59–74.
Bhoj, D.S. and Chandra, G. (2019). Simple unequal allocation procedure for ranked set sampling with skew distributions. Journal of Modern Applied Statistical Methods, 18(2), eP2811. doi: 10.22237/jmasm/1604189700.
Chandra, G., Bhoj, D.S. and Pandey, R. (2018). Simple unbalanced ranked set sampling for mean estimation of response variable of developmental programs, Journal of Modern Applied Statistical Methods, 17(1). Article 28. DOI: 10.22237/jmasm/1543856083.
Chandra, G., Nautiyal, R. and Chandra, H. (Eds.) (2020). Statistical methods and applications in forestry and environmental sciences, Springer, Singapore.
Chandra, G., Tiwari, N. and Chandra, H. (2011). Adaptive cluster sampling based on ranked sets. MetodoloskiZvezki (Advances in Methodology and Statistics), 8(1), 39-55.
Chandra, G., Tiwari, N. and Nautiyal, R. (2015). Near optimal allocation models for symmetric distributions in ranked set sampling. In Chandra, G., Nautiyal, R., Chandra, H., Roychoudury, N and Mohammad, N. (Eds.), Statistics in Forestry: Methods and Applications. Bonfring, Coimbatore. pp. 85-90.
Chandra, G., Tiwari, N. and Nautiyal, R. (2019). Two stage adaptive cluster sampling based on ordered statistics. Metodoloski Zvezki (Advances in Methodology and Statistics), 16(1), 43-60.
Cobby, J.M., Ridout, M.S., Bassett, P.J. and Large, R.V. (1985). An investigation into the use of ranked set sampling on grass and grass-clover swards. Grass and Forage Science, 40, 257-263.
Evans, M.J. (1967). Application of ranked set sampling to regeneration surveys in areas direct-seeded to longleaf pine. Masters Thesis, School of Forestry and Wildlife Management, Louisiana State University, Baton Rouge.
Grosenbaugh, L.R. (1963). Some suggestions for better sample-tree measurements. Soc. Am. Forest Proc. 36–42.
Grosenbaugh, L.R. (1965). Three-pee sampling theory and program ‘THRP’ for computer generation of selection criteria. U.S. For. Serv. Res. Pap. PSW-21.
Halls, L.K., and Dell, T.R. (1966). Trials of ranked set sampling for forage yields. Forest Science, 12, 22-26.
Harter, H.L. and Balakrishnan, N. (1996). CRC handbook of tables for the use of order statistics in estimation, CRC Press, Boca Raton, New York.
Hastings, C. Jr., Mosteller, F., Tukey, J.W. and Winsor, C.P. (1947). Low moments for small samples: A comparative study of order statistics. The Annals of Mathematical Statistics, 18(3), 413-426.
Iles, K. (2003). A sampler of inventory topics. 2nd ed. Kim Iles and Associates, Nanaimo, BC. 869 p.
Kaur, A., Patil, G.P. and Taillie, C. (1997). Unequal allocation models for ranked set sampling with skew distributions. Biometrics, 53, 123-130.
Kaur, A., Patil, G.P. and Taillie, C. (2000). Optimal allocation for symmetric distributions in ranked sampling. Annals of the Institute of Statistical Mathematics, 52(2), 239-254.
Kershaw, J. A., Jr., Ducey, M. J., Beers, T. W. and Husch, B. (2016). Forest Mensuration. 5th ed. Wiley/Blackwell, Hobokin, NJ. 640 p.
Kumar, A., Chandra, G. and Kumar, S. (2019). Estimating bark eating caterpillars Indarbelaquadrinotata (Walker) in Populous deltoides using ranked set sampling. Indonesian Journal of Applied Statistics, 3(1), 1-11.
Kvam, P.H. (2003). Ranked set sampling based on binary water quality data with covariates. Journal of Agricultural, Biological, and Environmental Statistics, 8, (3), 271–279.
Latpate, R. Kshirsagar, J., Gupta, V.K. and Chandra, G. (2021). Advanced Sampling Methods, Springer, Singapore. 301 p.
Martin, W.L., Sharik, T.L., Oderwald, R.G. and Smith, D.W. (1980). Evaluation of ranked set sampling for estimating shrub phytomass in Appalachian oak forests. Blacksburg, Virginia: School of Forestry and Wildlife Resources, Virginia Polytechnic Institute and State University, FWS, 4-80.
McIntyre, G.A. (1952). A method for unbiased selective sampling using ranked sets. Australian Journal of Agricultural Research, 3, 385-390.
Mode, N., Conquest, L. and Marker, D. (1999). Ranked set sampling for ecological research: Accounting for the total cost of sampling. Environmetrics, 10, 179–194.
Nelson, L.E., Switzer, G.L and Lockaby, B.G. (1987). Nutrition of Populus deltoides plantations during maximum production. Forest Ecology and Management, 20(1-2), 25–41.
Platt, W.J. Evans, G.W. and Rathbun, S.L. (1988). The population dynamics of a long-lived conifer Pinus palustris. American Naturalist, 131(4), 491–525.
Sarhan, A.E. and Greenberg, B.G. (eds.) (1962). Contributions to order statistics, John Wiley.
Tarr, A.B., Moore, K.J. Burras, C.L., Bullock, D.G. and Dixon, P.M. (2005). Improving map accuracy of soil variables using soil electrical conductivity as a covariate. Precision Agriculture, 6 (3), 255–270.
Thompson, S.K. (1990). Adaptive cluster sampling. Journal of the American Statistical Association, 85, 1050-1059.
Thompson, S.K. (2012). Sampling. 3rd ed. Wiley, New York. 472 p. (p. 147).
Tiwari, N. and Chandra, G. (2011). A systematic procedure for unequal allocation for skewed distributions in ranked set sampling. Journal of the Indian Society of Agricultural Statistics, 65(3), 331-338.
Wang, Y.G., Ye, Y. and Milton D.A. (2009). Efficient designs for sampling and subsampling in fisheries research based on ranked sets. ICES Journal of Marine Science, 66 (5), 928–934.
Yang, T.R., Kershaw, J.A., Jr., Weiskittel, A.R., Lam, T.Y. and McGarrigle, E. (2019). Influence of sample selection design and estimation method on sample size requirements for LiDAR-assisted forest inventories. Forestry. 92, 311–323.
Refbacks
- There are currently no refbacks.

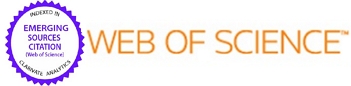
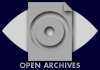
© 2008 Mathematical and Computational Forestry & Natural-Resource Sciences