Spatial Interpolation of Above-Ground Biomass in Labanan Concession Forest in East Kalimantan, Indonesia
Abstract
Keywords
Full Text:
PDFReferences
Basuki, T. M., P. E. van Laake, A. K. Skidmore, and Y. A. Hussin, 2009. Allometric equations for estimating the above-ground biomass in tropical lowland Dipterocarp forests. Forest Ecology and Management 257(8): 1684–1694.
Berau Forest Management Project, 2001. Mapping vegetation and forest types using Landsat TM in the Labanan concession of PT Inhutani I. Berau Forest Management Project, 16 pp.
Berthouex, P. M., and L. C. Brown, 2002. Statistics for environmental engineers. 2nd edition. Boca Raton, Lewis Publishers, 489 pp.
Blujdea, V. N. B., R. Pilli, I. Dutca, L. Ciuvat, and I. V. Abrudan, 2012. Allometric biomass equations for young broadleaved trees in plantations in Romania. Forest Ecology and Management 264: 172–184.
Brown, S., 1997. Estimating biomass and biomass change of tropical forests: A primer. FAO Forestry Paper 134. UN-FAO, Rome, Italy, 55 pp.
Castillo-Santiago, M. Ã., A. Ghilardi, K. Oyama, J. L. Hernández-Stefanoni, I. Torres, A. Flamenco-Sandoval, A. Fernández, and J. Mas, 2013. Estimating the spatial distribution of woody biomass suitable for charcoal making from remote sensing and geostatistics in central Mexico. Energy for Sustainable Development 17(2): 177–188.
De Jong, S. M., E. J. Pebesma, and B. Lacaze, 2003. Above-ground biomass assessment of Mediterranean forests using airborne imaging spectrometry: The DAIS Peyne experiment. International Journal of Remote Sensing 24(7): 1505–1520.
Diggle, P. J., and P. J. Ribeiro, 2007. Model-based geostatistics. New York, NY, Springer, 228 pp.
Djomo, A. N., A. Ibrahima, J. Saborowski, and G. Gravenhorst, 2010. Allometric equations for biomass estimations in Cameroon and pan moist tropical equations including biomass data from Africa. Forest Ecology and Management 260(10): 1873–1885.
ESRI (Environmental Systems Resource Institute), 2013. ArcMap 10.2. ESRI, Redlands, California.
Fortin, M. -J., and M. R. T. Dale, 2005. Spatial analysis: A guide for ecologists. Cambridge, UK, Cambridge University Press, 365 pp.
Freeman, E. A., and G. G. Moisen, 2007. Evaluating kriging as a tool to improve moderate resolution maps of forest biomass. Environmental Monitoring and Assessment 128(1-3): 395–410.
Galeana-Pizaña, J. M., A. López-Caloca, P. López-Quiroz, J. L. Silván-Cárdenas, and S. Couturier, 2014. Modeling the spatial distribution of above-ground carbon in Mexican coniferous forests using remote sensing and a geostatistical approach. International Journal of Applied Earth Observation and Geoinformation 30: 179–189.
Goovaerts, P., 1997. Geostatistics for natural resources evaluation. New York, Oxford University Press, 483 pp.
Hero, J. -M., J. G. Castley, S. A. Butler, and G. W. Lollback, 2013. Biomass estimation within an Australian eucalypt forest: Meso-scale spatial arrangement and the influence of sampling intensity. Forest Ecology and Management 310: 547–554.
Isaaks, E. H., and R. M. Srivastava, 1989. An introduction to applied geostatistics. New York, Oxford University Press, 561 pp.
Kitanidis, P. K., 1997. Introduction to geostatistics: Applications in hydrogeology. New York, Cambridge University Press, 249 pp.
Lamsal, S., D. M. Rizzo, and R. K. Meentemeyer, 2012. Spatial variation and prediction of forest biomass in a heterogeneous landscape. Journal of Forestry Research 23(1): 13−22.
Laurin, G. V., Q. Chen, J. A. Lindsell, D. A. Coomes, F. D. Frate, L. Guerriero, F. Pirotti, and R. Valentini, 2014. Above ground biomass estimation in an African tropical forest with lidar and hyperspectral data. ISPRS Journal of Photogrammetry and Remote Sensing 89: 49–58.
Lu, D., 2006. The potential and challenge of remote sensing-based biomass estimation. International Journal of Remote Sensing 27(7): 1297–1328.
Montes, F., and A. Ledo, 2010. Incorporating environmental and geographical information in forest data analysis: A new fitting approach for universal kriging. Canadian Journal of Forest Research 40(9): 1852–1861.
Pan, Y., R. A. Birdsey, J. Fang, R. Houghton, P. E. Kauppi, W. A. Kurz, O. L. Phillips, A. Shvidenko, S. L. Lewis, J. G. Canadell, P. Ciais, R. B. Jackson, S. W. Pacala, A. D. McGuire, S. Piao, A. Rautiainen, S. Sitch, and D. Hayes, 2011. A large and persistent carbon sink in the world’s forests. Science 333: 988–993.
Pearson, T. R. H., S. L. Brown, and R. A. Birdsey, 2007. Measurement guidelines for the sequestration of forest carbon. General Technical Report NRS-18. Newtown Square, PA: U.S. Department of Agriculture, Forest Service, Northern Research Station, 42 pp.
R Core Team, 2014. R: A language and environment for statistical computing. R Foundation for Statistical Computing, Vienna, Austria. URL http://www.R-project.org/.
Ribeiro, P. J., Jr., and P. J. Diggle, 2001. geoR: A package for geostatistical analysis. R-NEWS 1: 15–18.
Rutishauser, E., F. Noor’an, Y. Laumonier, J. Halperin, Rufi’ie, K. Hergoualc’h, and L. Verchot, 2013. Generic allometric models including height best estimate forest biomass and carbon stocks in Indonesia. Forest Ecology and Management 307: 219–225.
Sales, M. H., Souza, C. M., Jr., Kyriakidis, P. C., Roberts, D. A., and Vidal, E, 2007. Improving spatial distribution estimation of forest biomass with geostatistics: A case study for Rondônia, Brazil. Ecological Modeling 205: 221–230.
Samalca, I., 2007. Estimation of forest biomass and its error: A case in Kalimantan, Indonesia. Unpublished MSc. Thesis. ITC the Netherlands, Enchede, 84 pp.
Saatchi, S. S., R. A. Houghton, R. C. Dos Santos Alvalá, J. V. Soares, and Y. Yu, 2007. Distribution of aboveground live biomass in the Amazon basin. Global Change Biology 13(4): 816–837.
Schabenberger, O., and F. J. Pierce, 2002. Contemporary statistical models for the plant and soil sciences. Boca Raton, Florida, CRC Press LLC, 738 pp.
Tanase, M. A., R. Panciera, K. Lowell, S. Tian, J. M. Hacker, and J. P. Walker, 2014. Airborne multi-temporal L-band polarimetric SAR data for biomass estimation in semi-arid forests. Remote Sensing of Environment 145: 93–104.
Tiryana, T., 2005. Predicting spatial distribution of stand volume using geostatistics. Jurnal Manajemen Hutan Tropika 11(2): 15–27.
Tsui, O. W., N. C. Coops, M. A. Wulder, and P. L. Marshall, 2013. Integrating airborne LiDAR and space-borne radar via multivariate kriging to estimate above-ground biomass. Remote Sensing of Environment 139: 340–352.
Usuga, J. C. L, J. A. R. Toro, M. V. R. Alzate, and Ã. J. L. Tapias, 2010. Estimation of biomass and carbon stocks in plants, soil and forest floor in different tropical forests. Forest Ecology and Management 260(10): 1906–1913.
Wackernagel, H., 2003. Multivariate geostatistics: An introduction with applications. Berlin, New York, Springer, 387 pp.
Webster, R., and M. A. Oliver, 2007. Geostatistics for environmental scientists. 2nd edition. Chichester, Hoboken, NJ, John Wiley & Sons, Ltd., 315 pp.
Wijaya, A., S. Kusnadi, R. Gloaguen, and H. Heilmeier, 2010a. Improved strategy for estimating stem volume and forest biomass using moderate resolution remote sensing data and GIS. Journal of Forestry Research 21(1): 1–12.
Wijaya, A., V. Liesenberg, and R. Gloaguen, 2010b. Retrieval of forest attributes in complex successional forests of central Indonesia: Modeling and estimation of bitemporal data. Forest Ecology and Management 259(12): 2315–2326.
Zhang, J., S. Huang, E. H. Hogg, V. Lieffers, Y. Qin, and F. He., 2014. Estimating spatial variation in Alberta forest biomass from a combination of forest inventory and remote sensing data. Biogeosciences 11(10): 2793–2808.
Refbacks
- There are currently no refbacks.

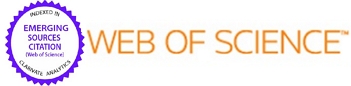
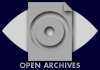
© 2008 Mathematical and Computational Forestry & Natural-Resource Sciences