Parameter Estimation And Data-Driven Method For Forest Fire Prediction
Abstract
Improvement in the accuracy of the forest fire prediction model is essential to properly instructfirefighting forces. The input parameters of traditional prediction method cannot be adjusted in real-time,so the forecasting accuracy will decrease over time. To solve this problem, the forest fire predictionsystembased on parameter estimation and data-driven method is proposed in this paper. First, twodynamic parameters based on the empirical formula, rate of fire spread and main spreading direction,and multi-sensor data are input to a forward prediction model based on the Huygens principle togenerate the predicted fireline for the current time. Secondly, the difference between the predicted andobserved firelines is minimized by the Grey Wolf Optimization algorithm, which derives the optimaldynamic parameters.Finally, the optimal parameters and the current multi-sensor data are input into theprediction model to achieve accurate prediction of the fireline. The burn experiment was designed, andthe feasibilityof the systemwasverifiedbyreal fire data. The results indicate thata fire prediction systemthat quickly calibrates dynamic input parametersis developed and can achieve real-time accurate firepredictions.
Keywords
Full Text:
PDFReferences
Altintas, I., Block, J., De Callafon, R., Crawl, D., Cowart, C., Gupta, A., Nguyen, M., Braun, H.-W., Schulze, J., Gollner, M., et al. (2015). Towards an integrated cyberinfrastructure for scalable data-driven monitoring, dynamic prediction and resilience of wildfires. Procedia Computer Science, 51:1633–1642.
Amoiralis, E. I., Tsili, M. A., Paparigas, D. G., and Kladas, A. G. (2014). Global transformer design optimization using deterministic and nondeterministic algorithms. IEEE Transactions on Industry Applications, 50(1):383–394.
Anderson, K., Reuter, G., and Flannigan, M. D. (2007). Fire-growth modelling using meteorological data with random and systematic perturbations. International Journal of Wildland Fire, 16(2):174–182.
Cardil, A., Monedero, S., Schag, G., de Miguel, S., Tapia, M., Stoof, C. R., Silva, C. A., Mohan, M., Cardil, A., and Ramirez, J. (2021). Fire behavior modeling for operational decision-making. Current Opinion in Environmental Science & Health, 23:100291.
Carrasco, J., Pais, C., Shen, Z.-J. M., and Weintraub, A. (2019). Adjusting rate of spread factors through derivative-free optimization: a new methodology to improve the performance of forest fire simulators. arXiv preprint arXiv:1909.05949.
Choi, B.-D., Han, J.-W., Kim, C.-S., and Ko, S.-J. (2006). Frame rate up-conversion using perspective transform. IEEE Transactions on Consumer Electronics, 52(3):975–982.
Denham, M., Wendt, K., Bianchini, G., Cort´ es, A., and Margalef, T. (2012). Dynamic data-driven genetic algorithm for forest fire spread prediction. Journal of Computational Science, 3(5):398–404.
Erkan, U., Gökrem, L., and Engino? glu, S. (2018). Different applied median filter in salt and pepper noise. Computers & Electrical Engineering, 70:789–798.
Ferragut, L., Asensio, M., Cascón, J., and Prieto, D. (2015). A wildland fire physical model well suited to data assimilation. Pure and Applied Geophysics, 172(1):121–139.
Finney, M. A. (1998). Farsite: Fire area simulator-model development and evaluation. USDA Forest Service Research Papers RMRS, (RP-4).
Griffiths, D. (1999). Improved formula for the drought factor in mcarthur’s forest fire danger meter. Australian Forestry, 62(2):202–206.
Lagarias, J. C., Reeds, J. A., Wright, M. H., and Wright, P. E. (1998). Convergence properties of the nelder– mead simplex method in low dimensions. SIAM Journal on optimization, 9(1):112–147.
Li, X., Zhang, M., Zhang, S., Liu, J., Sun, S., Hu, T., and Sun, L. (2022). Simulating forest fire spread with cellular automation driven by a lstm based speed model. Fire, 5(1):13.
Mandel, J., Beezley, J. D., Coen, J. L., and Kim, M. (2009). Data assimilation for wildland fires. IEEE Control Systems Magazine, 29(3):47–65.
Meng, Z. (2019). Survey on inverse problem and its inversion methods of impact dynamics. Science Technology and Engineering, 19(04):1–8.
Mirjalili, S., Mirjalili, S. M., and Lewis, A. (2014). Grey wolf optimizer. Advances in engineering software, 69:46–61.
Monedero, S., Ramirez, J., and Cardil, A. (2019). Predicting fire spread and behaviour on the fireline. wildfire analyst pocket: A mobile app for wildland fire prediction. Ecological Modelling, 392:103–107.
Norouzi, A., Rahim, M. S. M., Altameem, A., Saba, T., Rad, A. E., Rehman, A., and Uddin, M. (2014). Medical image segmentation methods, algorithms, and applications. IETE Technical Review, 31(3):199–213.
Richards, G. D. (1990). An elliptical growth model of forest fire fronts and its numerical solution. International journal for numerical methods in engineering, 30(6):1163–1179.
Richards, G. D. (1993). The properties of elliptical wildfire growth for time dependent fuel and meteorological conditions. Combustion science and technology, 95(16):357–383.
Rochoux, M. C., Delmotte, B., Cuenot, B., Ricci, S., and Trouv´ e, A. (2013). Regional-scale simulations of wildland fire spread informed by real-time flame front observations. Proceedings of the Combustion Institute, 34(2):2641–2647.
Rote, G. (1991). Computing the minimum hausdorff distance between two point sets on a line under translation. Information Processing Letters, 38(3):123–127.
Rothermel, R. (1972). A mathematical model for predicting fire spread in wildland fuels. Usda Forest Service General Technical Report, 115.
Srivas, T., de Callafon, R. A., Crawl, D., and Altintas, I. (2017). Data assimilation of wildfires with fuel adjustment factors in farsite using ensemble kalman filtering. Procedia Computer Science, 108:1572–1581.
Tolhurst, K., Shields, B., and Chong, D. (2008). Phoenix: development and application of a bushfire risk management tool. Australian journal of emergency management, 23(4):47–54.
Tymstra, C., Bryce, R., Wotton, B., Taylor, S., Armitage, O., et al. (2010). Development and structure of prometheus: the canadian wildland fire growth simulation model. Natural Resources Canada, Canadian Forest Service, Northern Forestry Centre, Information Report NOR-X-417.(Edmonton, AB).
Wang, D., Tan, D., and Liu, L. (2018). Particle swarm optimization algorithm: an overview. Soft computing, 22:387–408.
Wang, Z. (1983). The mesurement method of the wildfire initial spread rate. Mountain Research, 1(2):42–51. Whitley, D. (1994). A genetic algorithm tutorial. Statistics and computing, 4(2):65–85.
Yang, N., Qian, Y., EL-Mesery, H. S., Zhang, R., Wang, A., and Tang, J. (2019). Rapid detection of rice disease using microscopy image identification based on the synergistic judgment of texture and shape features and decision tree–confusion matrix method. Journal of the Science of Food and Agriculture, 99(14):6589– 6600.
Yu, J., Gao, N., Meng, Z., and Zhang, Z. (2021). Highaccuracy projector calibration method for fringe projection profilometry considering perspective transformation. Optics Express, 29(10):15053–15066.
Zhang, X., Liu, P., and Wang, X. (2020). Research on improvement of wang zhengfei’s forest fire spread model. Journal of Shandong Forestry Science and Technology, 50(01):1–6.
Refbacks
- There are currently no refbacks.

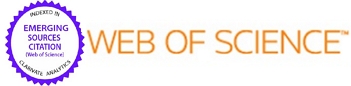
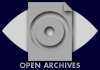
© 2008 Mathematical and Computational Forestry & Natural-Resource Sciences