Mapping natural forest stands with low cost drones
Abstract
Keywords
Full Text:
PDFReferences
Agisoft. 2019. Agisoft Metashape. St. Petersburg: Russia. Agisoft LLC.
Bechtold, W.A., and P.L. Patterson (eds.). 2005. The enhanced forest inventory and analysis program - national sampling design and estimation procedures. General Technical Report SRS-80. Asheville, NC: U.S. Department of Agriculture, Forest Service, Southern Research Station.
Csillik, O., J. Cherbini, R. Johnson, A. Lyons, and M. Kelly. 2018. Identification of citrus trees from unmanned aerial vehicle imagery using convolutional neural networks. Drones. 2: Article 39.
Dabberdt, W.F., D.H. Lenschow, T.W. Horst, P.R. Zimmerman, S.P. Oncley, and A.C. Delany. 1993. Atmosphere-surface exchange measurements. Science. 260: 1472-1481.
dos Santos, A.A., J.M. Junior, M.S. Araújo, D.R. Di Martini, E.C. Tetila, H.L. Siqueira, C. Aoki, A. Eltner, E.T. Matsubara, H. Pistori, and R.Q. 2019. Assessment of CNN-based methods for individual tree detection on images captured by RGB cameras attached to UAVs. Sensors. 19: Article 3595.
Guerra-Hernández J., E. González-Ferriro, V.J. Monleón, S.P. Faias, M. Tomé, and R.A. Diaz-Varela. 2017. Use of multi-temporal UAV-derived imagery for estimating individual tree growth in Pinus pinea stands. Forests. 8: Article 300.
Litchi. 2018. Litchi for DJI Mavic / Phantom / Inspitre / Spark. Brooksville, FL: VC Technology, Inc.
Novick, K.A., J.A. Biederman, A.R. Desai, M.E. Litvak, D.J.P. Moore, R.L. Scott, and M.S. Torn. 2018. The Ameriflux network: A collaboration of the willing. Agricultural and Forest Meteorology. 249: 444-456.
Onishi, M., and T. Ise. 2018. Automatic classification of trees using a UAV onboard camera and deep learning. arXiv: 1804.10390.
Salach A., K. Bakuła, M. Pilarska, W. Ostrowski, K. Górski, and Z. Kurczyński. 2018. Accuracy assessment of point clouds from LiDAR and dense image matching acquired using the UAV platform for DTM creation. ISPRS International Journal of GEO-Information. 7: Article 342.
Wallace L., A. Lucieer, Z. Malenovský, D. Turner, and P. Vopěnka. 2016. Assessment of forest structure using two UAV techniques: A comparison of airborne laser scanning and structure from motion (SfM) point clouds. Forests. 7: Article 62.
Wang, Y., H. Weinacker, B. Koch, and K. Stereńczak. 2008. LiDAR point cloud based fully automatic 3D single tree modelling in forest and evaluations of the procedure. The International Archives of the Photogrammetry, Remote Sensing and Spatial Information Sciences. XXXVII(Part B6b): 45-51.
Williams B.J., Williams T.M. O’Halloran T.L., and Song B. 2018. Comparison of low cost UAVs to accurately measure tree size and location. P 47-58 In Merry K, Bettinger P., Cieszewski C, Crosby M, Lowe R, Siry J. Proceedings 11th Southern Forestry and Natural Resource Management GIS Conference . University of Georgia, Athens GA, Dec 11-12, 2017
Refbacks
- There are currently no refbacks.

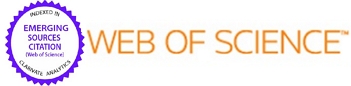
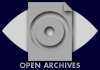
© 2008 Mathematical and Computational Forestry & Natural-Resource Sciences