Optimizing the Rothermel model for easily Predicting spread rate of forest fire
Abstract
Keywords
Full Text:
PDFReferences
Dhall, A., Dhasade, A., Nalwade, A. and Kulkarni, V. 2020. A survey on systematic approaches in manag- ing forest fires. Applied geography, 121:102266. DOI:- 10.1016/j.apgeog.2020.102266
Brun, C., Art´ es, T., Cencerrado, A., Margalef, T. and Cort´ es, A. 2017. A high performance computing framework for continental-scale forest fire spread pre- diction. Procedia Computer Science, 108:1712-1721. DOI:10.1016/j.procs.2017.05.258.
Ding Z., Song K., Wang Z. 2014. Ontrastive analysis of algorithms of the forest fire spreading simulation based on Arc GIS Engine. Journal of University of Chinese Academy of Sciences. 31(5):640-646. DOI:- 2095-6134(2014)05-0640-07.
Ervilha, A. R., Pereira, J. M. C. and Pereira, J. C. F. 2017. On the parametric uncertainty quan- tification of the Rothermel’s rate of spread model. Applied Mathematical Modelling, 41:37-53. DOI:- 10.1016/j.apm.2016.06.026.
Gigovic, L., Pourghasemi, H. R., Drobnjak, S. and Bai, S. 2019. Testing a new ensemble model based on SVM and random forest in forest fire susceptibility assessment and its mapping in Serbia’s Tara National Park. Forests, 10(5):408. https://doi.org/10.3390/f10050408.
Lopes A. M. G., Ribeiro. 2019. Simulation of forest fire spread using a two-way coupling algorithm and its ap- plication to a real wildfire. Journal of Wind Engineer- ing and Industrial Aerodynamics, 193:103967. DOI:- 10.1016/j.jweia.2019.103967.
Li D., Jin J. 2104. Study on errors of forest respread rate measured by visible light image method. Journal of Central South University of Forestry and Technology. 34(3):61-67.
Li, X., Gao, H., Han, C., Wang, Y., Hu, T., Sun, L., and Guo, Y. (2020). An optimized model for predicting forest fires area based on binocular vision. Mathemat- ical and Computational Forestry & Natural-Resource Sciences (MCFNS), 12(1):16-25. Retrieved from http://mcfns.net/index.php/Journal/article/view/12.2 on Oct. 2020. DOI:10.3969/j.issn.1673-923X.2014.03- .012
Man, Z., Sun, L., Hu, H., and Zhang, Y. 2019. Prediction Model of the Spread Rate of Eight typical Surface Dead Fuel in Southern China under Windless and Flat Land. Scientia Silvae Sinicae. 55 (7):197-204. DOI:- 10.11707/j.10017488.20190722.
Pan, D., Zhang, H., Pan, G., Yi, L. 2017. Re- search on masson pine potential fire behavior in southern subtropical based on Behave Plus. Jour- nal of Central South University of Forestry and Technology. 37 (06):14-23. DOI:10.14067/j.cnki.1673- 923x.2017.06.003.
Prince D., Shen C., Fletcher T. 2017. Semi-empirical model for fire spread in shrubs with spatially-defined fuel elements and flames. Fire Technology, 53 (3):1-31. DOI:10.1007/s10694-016-0644-9.
Rios, O., Pastor, E., Valero, M. M. and Planas, E. 2016. Short-term fire front spread prediction using in- verse modelling and airborne infrared images. Inter- national Journal of Wildland Fire, 25(10):1033-1047. DOI:10.1071/WF16031
Rossi, L., Molinier, T., Akhloufi, M., Pieri, A. and Ti- son, Y. 2013. Advanced stereovision system for fire spreading study. Fire safety journal, 60:64-72. DOI:- 10.1016/j.firesaf.2012.10.015.
Viegas, D. X., Almeida, M., Miranda, A. I. and Ribeiro, L. M., 2010.Linear model for spread rate and mass loss rate for mixed-size fuel beds. Interna- tional Journal of Wildland Fire, 19(5):531-540. DOI:- 10.1071/WF09007.
Dahl, N., Xue, H., Hu, X. and Xue, M. 2015. Cou- pled fire–atmosphere modeling of wildland fire spread using DEVS-FIRE and ARPS. Natural Hazards, 77(2):1013-1035. DOI:10.1007/s11069-015-1640-y.
Ying, L., Han, J., Du, Y. and Shen, Z. 2018. Forest fire characteristics in China: Spatial patterns and deter- minants with thresholds. Forest ecology and manage- ment, 424:345-354. DOI:10.1016/j.foreco.2018.05.020.
Yang, Z., Zhang, H., Zhang, L. and Chen, H. 2018. Experimental study on downslope fire spread over a pine needle fuel bed. Fire technology, 54(6):1487-1503. DOI:10.1007/s10694-018-0740-0.
Zhong Z., Huang W., Li S. 2017. Forest fire spread sim- ulating model using cellular automaton with extreme learning machine. Ecological Modelling. 348(12):33- 43. DOI:10.1016/j.ecolmodel.2016.12.022.
Zhang J., Liu B., Chu T. 2012. Fire behavior of ground surface fuels in Pinus koraiensis and Quercus mon- golica mixed forest under no wind and zero slope con- dition: A prediction with extended Rothermel model Chinese Journal of Applied Ecology. 23(6):1495-1502. DOI:1001-9332(2012)06-1495-08.
Refbacks
- There are currently no refbacks.

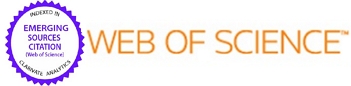
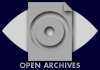
© 2008 Mathematical and Computational Forestry & Natural-Resource Sciences