Kriging with External Drift in Model Localization
Abstract
When a large-area model is utilized in smaller sub-areas, the results may be biased, even though the model is unbiased in general. One method for adjusting the large-area models for such bias is kriging, in which the predictions are corrected with the help of neighbouring observations. A variogram represents the spatial correlation between neighbouring observations as a function of distance. With the selected variogram and drift model that describes the general mean, the variable values for given objects are then predicted. The aim of this study was (1) to test for a spatial correlation in the residuals of a global form height model fitted over a large study area and (2) to use this correlation in prediction of the same variable. The dataset consisted of 19 175 Scots pines (Pinus sylvestris L.) from the 9th National Forest Inventory of Finland. Nested spherical and Bessel variograms were selected for the kriging calculations. In nested models the short-range intrastand correlation and long-range correlation are modelled separately. We used 10-fold cross-validation to evaluate the variogram models selected. We limited the number of neighbours from 20 to 100, i.e. at distances within an 8-17-km radius. At the global level, 30 neighbours were needed for stable estimates, and with 60 neighbours the RMSEs of kriging were lower than the globally fitted model. At the regional level, we obtained better estimates than with regionally re-fitted models when the number of neighbours was 60 for both variogram models. The biases at the regional level in the kriging were small (0.8% of the regional RMSE). In conclusion, there was an app. 6-km spatial correlation in the residuals, but the size of the kriging neighbourhood required for improving prediction was larger than the range. MCFNS 3(1):1-14.
Keywords
Full Text:
PDFRefbacks
- There are currently no refbacks.

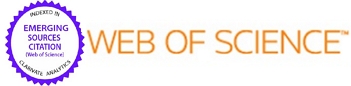
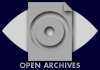
© 2008 Mathematical and Computational Forestry & Natural-Resource Sciences