Object-based forest cover change mapping using remote sensing in Nuristan Province, Afghanistan -- Poster Summary
Abstract
Deforestation and forest degradation are among significant environmental issues in Afghanistan but has not been studied intensively due to insecurity, confined budget, lack of expertise, and limited accessibility to new technology. In such a situation, remote sensing technology offers practical and economical means to acquire reliable, consistent, and up-to-date information for assessing forest cover and monitoring its spatial and temporal dynamics, especially in areas where field data collection is nearly impossible. Therefore, this study aims to detect deforestation in six districts of Nuristan Province over the past three decades using object-based classification of Landsat imagery. The specific objectives to achieve the overall objective of this study are: (i) ascertaining the current status of the forest cover, (ii) mapping forest cover in 1998, 2008, and 2016, and (iii) detecting forest cover change between 1988-2008 and 2008-2016. Overall, this study can be utilized as a baseline data of forest cover and its spatial and temporal dynamics of Nuristan Province, where the effort was to exploit the potential of freely available, medium-resolution Landsat data series that might contribute in future forest management, restoration, and conservation at a local, provincial, and national level in Afghanistan.
This study utilized the Landsat satellite imageries acquired from United States Geological Survey (USGS) for the years 1998, 2008, and 2016 to produce a new set of land cover (1998, 2008 and 2016) and forest cover change (1998-2008 and 2008-2016) maps for the six districts of Nuristan Province based on the use of a consistent set of methods and datasets using TNTmips Pro 2016 and eCognition Developer 9.0 softwares. The land cover maps were used to depict the land cover areas and transitions within each year, whereas the forest cover change maps indicated the extent of forest loss (deforestation) and forest gain (forest recover) together with the land and forest cover dynamics between two successive years or time-series i.e. (1998 and 2008) and (2008 and 2016) using object-based classification approach. This combination was in order to estimate the forest loss and gains, produce accurate and stable classification results, and reduce the year-to-year inconsistencies through the use of adequate numbers of training sample data. Meanwhile, the current study attempted to characterize the forest cover mapping based on its types. Overall, the research methodology comprises of (i) preprocessing of Landsat images using TNTmips (ii) object-based image classification using eCognition Developer 9.0, (iii) mapping land and forest cover change, and (iv) quantifying land cover dynamics together with forest cover loss and gains. Altogether, 13 main classes were assigned in the land cover maps with the help of hyperspectral SPOT-7 images and Google and Bing maps, whereas 3 additional change classes were added in the forest cover change maps (i) forest loss, (ii) forest gain, and (iii) seasonal snow cover.
Overall, the time series land cover mapping (1998, 2008, 2016) revealed that the dense broad-leaved forests have decreased (164.89 km2) from 1998 until 2016, whereas the sparse broad-leaved forests increased gradually within the same time duration. The increase (134.95 km2) in sparse broad-leaved forests during the study period was primarily attributed to a shift from dense broad-leaved forests to sparse broad-leaved forests, which can be defined as “forest degradationâ€. Furthermore, between 1998 and 2008, a large area of sparse broad-leaved forests have also been degraded and converted to other vegetation class which is not considered as forest. On the other hand, the district-based forest comparisons showed that Nurgeram district contained the highest amount of the forests (48.9% in 1998, 43.92% in 2008, and 45.24% in 2016) whereas, Parun district contained the lowest coverage of the forests (12.25% in 1998, 9.86% in 2008, and 11.07% in 2016) within the study area.
In addition, the findings from the forest cover change maps revealed that deforestation did not occur in a large and alarming quantity (7.26 km2) in the study area from 1998 to 2016. The results showed that the forest loss was 5.92 km2 in 1998-2008 change map, whereas forest gain was only 0.31 km2. In contrast, forest loss was decreased (1.34 km2) in 2008-2016 change map with surprisingly increase in forest area (1.24 km2). Although deforestation has not occurred in a large scale, the results illustrated that the forests have been continuously degraded during the study period, converting from dense broad-leaved forest to sparse as well as sparse to other vegetation areas.
Overall, the performed accuracy assessments on the final land cover and forest cover change maps showed that the utility of high-resolution images for choosing a sufficient number of training samples in Landsat images resulted in relatively high overall accuracies (>91 %). The maps indicated that most of the land cover classes have been better distinguished after applying the classification algorithms on the segmented images. However, some inconsistencies and misclassifications between the classes were encountered during data analysis, error matrices evaluation, and visual interpretation of the generated maps, where some are highlighted and exemplified as follows. Notably, the land cover and forest cover change maps failed to detect the settlement areas precisely (< 0.39 km2), which can be assumed due to the inadequate resolution of Landsat imagery in the identification of smaller areas, topographical features, and characteristics of the settlements. Moreover, some confusions were observed in the mixed forests with dense broad-leaved and coniferous forests, which were mainly assumed to be due to the dominance of coniferous or broad-leaved species within the mixed forests. Since a field survey was not implemented in this study, it is difficult to predict the main driving factors of forest degradation and deforestation in the area. Therefore, it is highly recommended that further research should be undertaken to analyze and explain the findings of this study in the context of underlying driving forces of change and assessing the impact on forest dynamics in Nuristan Province. Overall, such findings can be utilized to create a better understanding of forest conditions for the previous decades and provide sufficient and reliable information to decision makers and scientists to introduce sustainable management plans and implement concrete actions for forest restoration and conservation of natural forests at local, regional, and national levels.
Keywords
Full Text:
PDFRefbacks
- There are currently no refbacks.

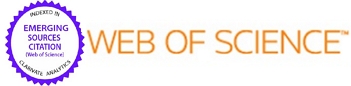
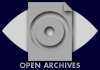
© 2008 Mathematical and Computational Forestry & Natural-Resource Sciences